
Let and denote the PGT images obtained using ( 3) and ( 4), respectively. We first analysed the proposed DnCNN trained using the PGT images. For guided image filtering in ( 4), the parameters r and were empirically chosen as 2 and 0.001, respectively.

However, we consider that 8-bit precision is more suitable for practical image post-processing applications. We used 8-bit precision for all images (, ,, and ), resulting in about 0.2 dB drop of the peak signal-to-noise ratio (PSNR) compared to the case in which floating-point precision was used. , 25, and 50, were tested, and the network was trained for each noise level individually . We thus used an enlarged training database consisting of image patches randomly cropped from the first 40,000 images of the Microsoft COCO 2017 training database however, the number of image patches used during training was set to be the same as that in the original DnCNN . ) were finally obtained using ( 3) or ( 4). ) were obtained from, and PGT images (i.e. After training the DnCNN, initially denoised images (i.e. ) in advance by applying the AWGN to GT images (i.e. Unlike the conventional implementation in which noisy images are dynamically synthesised during training, we generated noisy images (i.e.
DENOISE PHOTOS ONLINE CODE
In our experiment, we mainly used the author-provided source code of DnCNN . As the PGT image can still contain noise, we measure the loss from GT and PGT and control their weight using the parameter. Note that we maintain the DnCNN architecture and change only the loss function. (5)where is the augmented training database including the PGT images. Therefore, we try to reduce the distortion of denoised images from original images using the standard L2-loss loss function. In light of the perception-distortion tradeoff, we focus on applications requiring high fidelity to GT images. BackgroundĪs a proof of concept, we adopt one of the most widely used image denoising network architectures, called the denoising CNN (DnCNN) , as our baseline network and apply our method to the classic Gaussian denoising task. We experimentally verify that a denoising network that is trained to predict both PGT and GT images produces denoised images closer to GT images. We propose generating pseudo-ground-truth (PGT) images using original GT images and initially denoised images. This Letter shows that the performance of existing image denoising methods can be further improved without changing network architectures. For applications requiring high fidelity to GT images, such as medical, military, and security applications, de facto standard loss functions, i.e. However, the perception-distortion tradeoff was reported recently specifically, state-of-the-art GAN-based image restoration methods can only improve the perceptual image quality at the expense of distortion. The combination of perceptual loss and adversarial loss is very effective for reconstructing images with high perceptual quality . Adversarial loss originating from the generative adversarial network (GAN) is also used in many image restoration methods . Perceptual loss , a measure of the distance between feature maps extracted by the VGG network , is now widely used. Therefore, many alternative loss functions such as L1-loss, structural similarity index (SSIM), and multi-scale SSIM (MS-SSIM) have been investigated for image denoising . However, L2-loss is well known to correlate poorly with the perceptual image quality . One of the most widely used distortion measures is to directly compare pixel values of denoised and GT images using L2-loss.
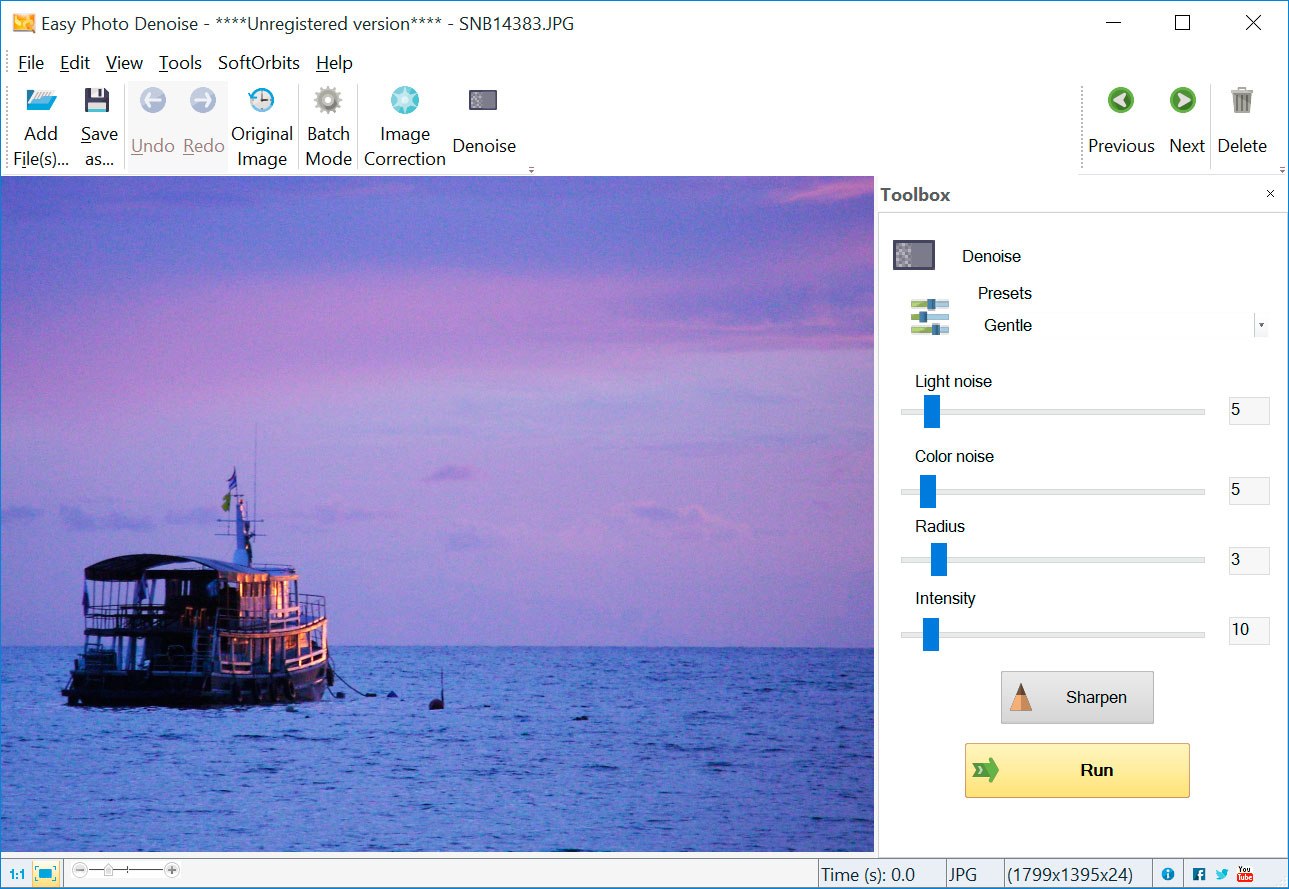
difference between denoised and GT images) can be minimised. As a large number of training images can be readily obtained by adding noise to ground-truth (GT) images, a deep CNN can be trained such that distortion (i.e. Owing to the success of deep learning techniques, especially convolutional neural networks (CNNs), discriminative approaches have become very popular for image denoising. Therefore, image denoising has become one of the most extensively studied topics in image processing. It degrades the visual quality and hampers computer vision tasks. Noise is unavoidable in tasks such as image capturing, compression, and transmission.

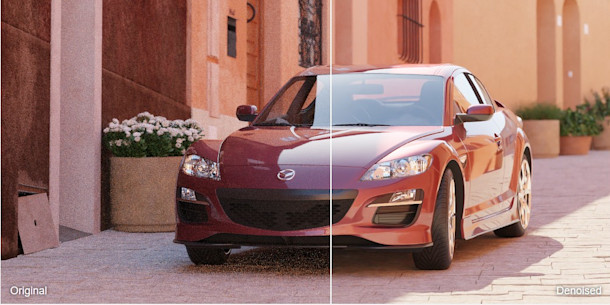
IET Electrical Systems in Transportation.IET Cyber-Physical Systems: Theory & Applications.IET Collaborative Intelligent Manufacturing.CAAI Transactions on Intelligence Technology.
